Harnessing Statistical Signal Processing for the Future of Passive Radar
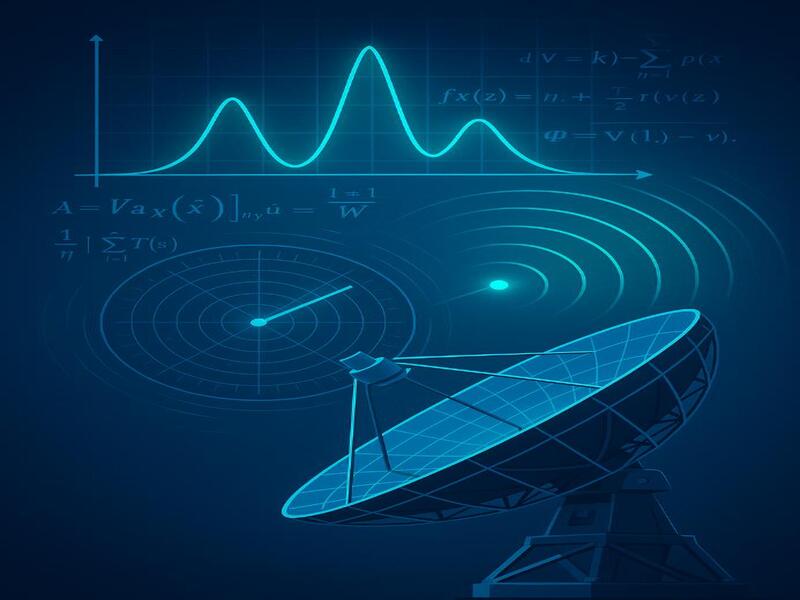
Imagine detecting aircraft or drones without ever emitting a single signal. Passive radar, an innovation transforming modern surveillance, makes it possible.
Unlike traditional radar systems that emit their own signals, passive radar leverages existing electromagnetic emissions—such as commercial broadcast and communication signals—to detect and track objects. This approach offers advantages like reduced cost, enhanced stealth, and the ability to operate in environments where active emissions are undesirable.
Statistical signal processing—where probability theory meets signal analysis—lies at the core of passive radar's remarkable capabilities. This blog post delves into recent advancements in statistical signal processing for passive radar and highlights how our department at Presidency University is at the forefront of this exciting domain.
Recent advances in deep learning have significantly enhanced radar signal processing. A recent study introduced a micro-Doppler coherence loss function in deep learning models, enhancing their robustness against noise. This advancement allows for more accurate activity classification and improved performance in real-world scenarios where radar signals are often obscured by noise.
The fusion of statistical signal processing and passive radar technology represents a frontier of innovation with vast potential applications—from defense to civilian sectors. At Presidency University, the Department of Electronics and Communication Engineering is committed to staying at the cutting edge of technological advancements, and students can position themselves at the nexus of this exciting field, ready to contribute to the next wave of technological breakthroughs.
Dr. Mohammad Shahnawaz Hussain, Assistant Professor,
Department of Electronics and Communication Engineering,
Presidency University, Bangalore